REFLECTING ON MARCH MADNESS 2024: AN ODE TO OUR PREDICTIVE MODEL'S MISADVENTURES
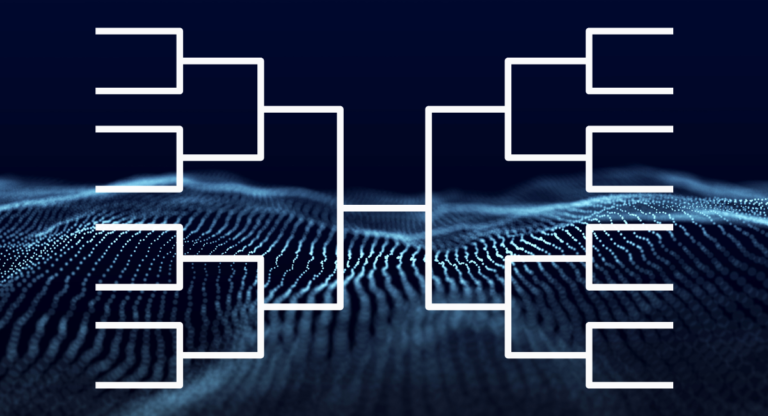
Written By: Chet Hayes, Vertosoft CTO
Oh, March Madness! That exhilarating time of year when college basketball reaches its zenith, and fans across the nation are glued to their screens, brackets in hand, hoping against hope that this year, they’ve cracked the code. And in my corner of the world, I had more than just hope; I had data. Or so I thought.
The Best Laid Plans of Mice and Models
Following the excitement around March Madness 2024, I was confident the advanced analytics model would led me to the office pool domination. Armed with machine learning algorithms, historical performance data, and what I believed was a solid strategy, I set out to conquer the courts of prediction.
However, as the games unfolded, it became glaringly apparent that the model’s performance was… let’s just say, less than stellar. By the Sweet 16, the model’s accuracy was rivaled only by that of a coin flip. By the Final Four, I am were pretty sure the coin was outperforming the model.
Where Did I Go Wrong? A Comedy of Errors
In the aftermath of my predictive debacle, I took a step back to assess where things went awry. And oh, the insights we gleaned were as enlightening as they were embarrassing:
- Data Quality Matters: It turns out, using player performance data from neighborhood pickup games doesn’t quite correlate to NCAA tournament outcomes. Who knew? – Ok, so it wasn’t quite that bad, but when the source of data had errors, it led to the following observation.
- Garbage In, Garbage Out: Feeding the model with team stats that were inaccurate, and erroneously calculated (Garbage In) resulted in some pretty bad results Garbage Out).
- Data Governance, Or Lack Thereof: My laissez-faire approach to data governance led to the wild west of stats, and I could have used “mascot fierceness index” and the “coach’s sideline wardrobe choice” and probably reached the same results.
Laughing My Way to Better Data Practices
In the spirit of learning (and a healthy dose of self-deprecation), I’m taking these lessons to heart. Here’s how I’m turning my blunders into building blocks for the future:
- Enhancing Data Quality: I am now as meticulous about my data sources as a cat is about its grooming habits. Only the most relevant, high-quality data will do.
- Implementing Robust Data Governance: No more cowboy coding. I have implementing strict data governance policies to ensure that every variable and model undergoes rigorous scrutiny (goodbye, mascot fierceness index).
My misadventures with the model taught me some invaluable lessons about the importance of data quality, governance, and the unpredictable nature of college basketball. So, here’s to better data, smarter models, and embracing the madness. May your brackets be ever in your favor, and may your data always be clean and well-governed.